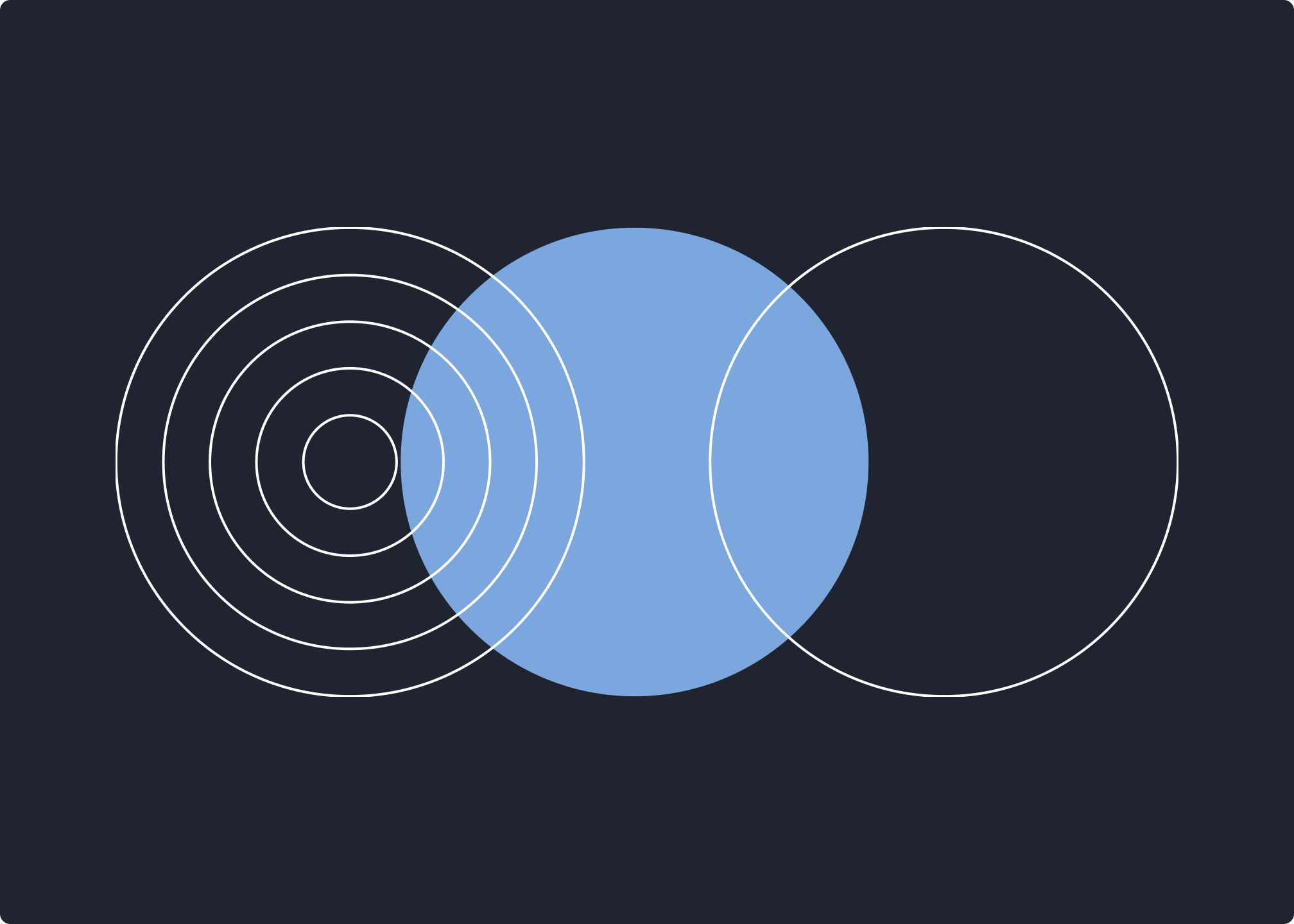
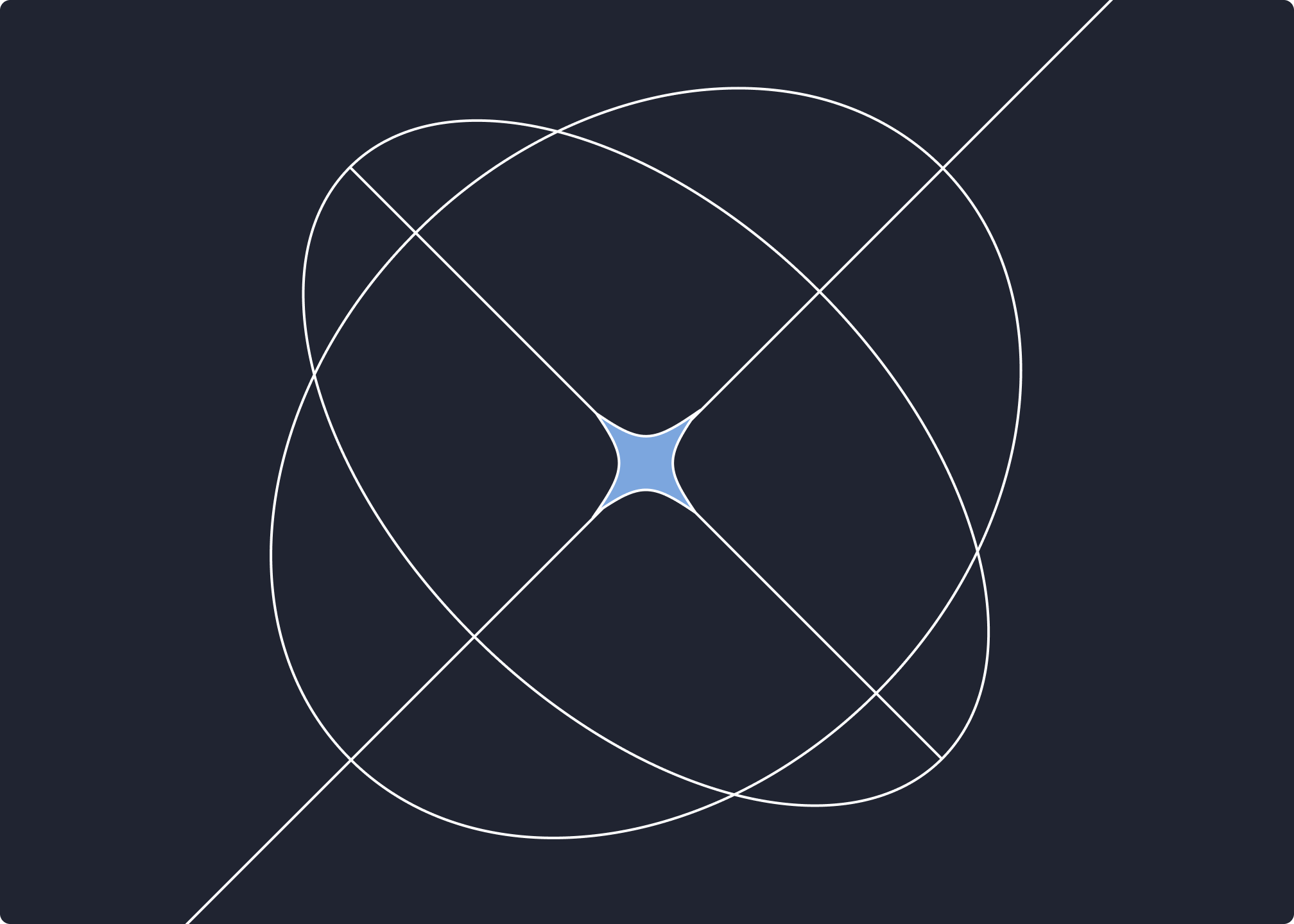

The objective of marketing has always been to show the right content to the right person at the right time. Historically, this ambition faced limitations due to manual segmentation, inconsistent user data, and limited delivery mechanisms. With the proliferation of digital touchpoints, achieving contextual relevance became even harder.
Traditional approaches rely heavily on rules-based personalization and static audience segmentation, where users are grouped into broad categories based on predefined criteria. These techniques lack the flexibility and granularity needed to engage today’s dynamic, always-on consumer.
Such systems often depend on historical averages and basic conditional logic, which fail to adapt to real-time behavioral signals or content performance feedback. The following limitations emerge:
To overcome these shortcomings, organizations need real time hyper-personalized adaptive systems that incorporate machine learning models, feedback mechanisms, and content intelligence engines to provide hyper-relevant, scalable, and cohesive user experiences across the customer journey.
Enters AI-powered Adaptive Content Optimization (ACO), a paradigm shift that merges real-time decision-making with machine learning to transform how content is created, delivered, and optimized across channels. This approach enables:
With adaptive content optimization, marketing becomes not only automated but also intelligent, iterative, and intimately connected to user intent and journey stage—enabling performance marketing at scale.
This new paradigm uses a suite of AI/ML models to detect intent, predict outcomes, and deliver dynamically composed experiences in real-time. Intent detection is powered by natural language understanding (NLU) and behavioral modeling using Transformer-based architectures (e.g., BERT, RoBERTa) which parse user interactions and classify them into specific intents or emotional states. Outcome prediction leverages supervised learning models such as Gradient Boosted Trees (e.g., XGBoost, LightGBM) and deep neural networks to score user actions like click-throughs or conversions with high precision.
For content generation and orchestration, reinforcement learning agents (e.g., Deep Q-Networks, Proximal Policy Optimization) continually adjust creative strategies based on real-time performance signals. Dynamic content assembly is guided by ranking models and contextual bandits, which balance exploration of new content with exploitation of known high-performing variants. These models are deployed in production using scalable inference platforms like TensorFlow Serving or ONNX Runtime, enabling millisecond-level decision-making.
Together, these systems enable marketers to algorithmically compose and serve content variants in response to each user’s real-time behavior, contextual conditions, and stage in the conversion funnel, creating a truly adaptive and responsive digital experience.
Let us look at two case studies of how we leveraged Machine Learning and Gen AI to deliver Hyper Personalized digital experiences through our Co-Marketer Platform to our end clients.
Case Study 2.1: NeutoAI’s AI-Driven Personalization Boosts Sales for a Leading Fashion Retailer
Client Overview
A well-known online fashion retailer faced high cart abandonment, low engagement, and declining conversion rates, despite a broad product catalog.
They engaged NeutoAI to implement an AI-driven solution that dynamically optimized content and created a hyper-personalized shopping experience.
GC developed and deployed CoMarketers, an advanced AI-powered personalization engine tailored to the client’s needs.
Solution & Implementation
1. AI-Driven User Behavior Tracking
2. Real-Time Personalization at Scale
When Jen returned to the site, the homepage dynamically updated to showcase:
3. AI-Powered Email & Push Notification Optimization
GC’s AI detected that Jen engages with emails in the evening and optimized messaging accordingly. She received a personalized email at 7 PM featuring:
📩 Subject Line: “Jen, Your Perfect Sneakers Are Waiting!”
🎯 A carousel of dynamic product recommendations based on her past searches.
💰 A special offer: “Complete Your Look – 10% Off Running Jackets for the Next 24 Hours!”
4. Real-Time Adjustments Based on Engagement
If Jen clicked on a product but didn’t purchase, the AI dynamically adapted her shopping experience:
🔹 AI-powered chat assistance recommended similar styles based on her preferences.
🔹 A pop-up alert created urgency: “Only 3 left in stock! Grab yours now.”
🔹 Social proof notifications boosted confidence: “150+ people bought this in the last 24 hours.”
Results & Impact
📈 28% increase in funnel conversion rates over 6 months.
🛒 20% reduction in cart abandonment after personalized retargeting efforts.
📧 35% higher engagement with AI-personalized emails compared to generic campaigns.
By implementing NeutoAI’s AI-powered dynamic content optimization, the retailer transformed its digital shopping experience—ensuring every customer interaction was timely, relevant, and conversion-driven. 🚀
Case Study 2.2: NeutoAI’s AI-Driven Personalization Enhances Telecom Plan Selection
Client Overview
A prominent telecom provider approached NeutoAI with challenges including low online conversions, frequent user drop-offs, and decreased engagement, despite offering an extensive range of telecom plans. To address these challenges, they partnered with NeutoAI to deploy an advanced AI-driven personalization strategy designed to dynamically optimize content and deliver a highly personalized user experience.
GrowthClap developed and implemented CoMarketers, a sophisticated AI-powered personalization engine tailored specifically to the telecom provider’s unique needs.
Solution & Implementation
1. AI-Driven User Behavior Tracking
A user, Sharad, visited the telecom website and explored unlimited data plans but exited without subscribing. Sharad, during his visit to the website, also reviewed family plans and compared various international calling options, demonstrating clear intent but not finalizing his decision.
GrowthClap’s AI system captured Sharad’s browsing behaviors, plan preferences, and potential purchase intentions in real time.
2. Real-Time Landing Page Personalization at Scale
Upon Sharad’s return to the telecom website, the homepage was dynamically adjusted through CoMarketers to display:
3. AI-Powered Email & Push Notification Optimization
Leveraging CoMarketers’ AI analytics, NeutoAI determined that Sharad typically engages more actively with marketing emails during morning hours.
4. Real-Time Adjustments Based on Engagement
If Sharad interacted with a product but still did not complete the purchase, CoMarketers immediately adjusted his experience:
🔹 An AI-powered chatbot proactively offered Sharad a personalized plan quiz to match telecom services accurately with his usage patterns.
🔹 A strategically timed limited-time pop-up appeared, stating: “Exclusive: 20% off your first 3 months if you switch today!” to encourage immediate action.
🔹 Social proof notifications reassured his choice by displaying messages like “5,000+ users switched to this plan last month!”, increasing the likelihood of purchase.
Results & Impact
📈 Significant increase in online plan conversions due to highly relevant personalized interactions.
🛒 Reduced abandonment rate after deployment of targeted real-time retargeting strategies.
📧 Higher email engagement, with substantial improvements compared to generic email campaigns.
By implementing NeutoAI’s sophisticated AI-powered dynamic content optimization, the telecom provider substantially improved user experience, ensuring each interaction was personalized, timely, and conducive to conversion. 🚀
These are prime examples of AI-driven hyper-personalization, ensuring end customers are presented with the right content at the right time based on their expressed or latent needs.
In this article, We will dive deeper to understand how businesses can craft highly targeted campaigns, enhance customer experiences, and maximize return on investment (ROI) by leveraging Machine learning, Natural language processing (NLP), and Real-time data analytics.
1️⃣ Advertising & Marketing Campaigns
Use Case: AI-driven A/B testing dynamically adjusts ad creatives, headlines, and CTAs based on real-time engagement.
Example: A digital ad campaign automatically switches to a high-performing version when click-through rates (CTR) drop below a threshold.
2️⃣ E-Commerce & Product Recommendations
Use Case: Personalized shopping experiences with dynamically optimized product pages.
Example: An online retailer adjusts homepage banners and product recommendations based on browsing behavior and past purchases.
3️⃣ Email & Push Notification Personalization
Use Case: AI optimizes subject lines, content, and send times to maximize open rates and engagement.
Example: A travel app sends personalized hotel deals based on a user’s recent searches and preferences.
4️⃣ Content Streaming & Media Personalization
Use Case: Platforms adapt content based on viewing behavior and engagement patterns.
Example: A music streaming app dynamically updates playlists based on listening habits, time of day, or mood.
5️⃣ Website & Landing Page Optimization
Use Case: Real-time content customization based on visitor behavior and demographics.
Example: A B2B SaaS company dynamically adjusts case studies shown on the website based on industry and user location.
6️⃣ Conversational AI & Chatbots
Use Case: AI-powered chatbots modify their responses based on user sentiment, past interactions, and browsing history.
Example: An AI assistant for a telecom company adapts its responses to recommend personalized phone plans.
7️⃣ Retail In-Store Digital Displays
Use Case: Adaptive digital signage changes promotions and content based on customer demographics and store traffic patterns.
Example: A clothing store display showcases relevant outfits based on local weather conditions and sales trends.
8️⃣ Customer Support & Knowledge Base Optimization
Use Case: AI dynamically adjusts FAQs and suggested help articles based on user queries.
Example: A software company’s help center prioritizes troubleshooting guides that match user behavior and past support tickets.
9️⃣ Social Media Content Optimization
Use Case: AI adapts post formats, hashtags, and imagery based on audience engagement trends.
Example: A fashion brand’s Instagram posts are automatically optimized based on trending styles and user engagement.
ACO is powered by a synergy of advanced AI/ML components and infrastructure, enabling real-time, hyper-personalized content experiences at scale:
Together, these components form a robust adaptive content pipeline capable of ingesting real-time signals, making intelligent decisions at millisecond latency, and delivering contextually aware experiences that evolve continuously with user behavior and business objectives.
Before we explore each architectural component in depth, it’s important to understand the overarching objective of the ACO architecture: to seamlessly connect real-time user data with decision engines, dynamic content delivery systems, and adaptive learning mechanisms in a way that is both scalable and responsive.
At a high level, the ACO architecture is structured to process vast streams of data—both historical and real-time—using a combination of machine learning pipelines, streaming data frameworks, and API-based integrations. These layers work in concert to:
The architecture is modular and highly extensible, supporting integration with third-party marketing stacks, analytics platforms, content management systems, and experimentation tools.
The following sections provide a detailed breakdown of each architectural layer and the AI/ML models, technologies, and business logic underpinning them.
5.1. Data Integration and Data Management Layer
The Data Integration and Management Layer serves as the foundational backbone of any Adaptive Content Optimization (ACO) architecture. It is responsible for capturing, normalizing, enriching, and transforming a variety of high-volume, high-velocity data streams from digital sources into structured representations that power downstream machine learning pipelines and personalization engines.
This layer combines best practices from modern data engineering—such as event-driven stream ingestion, data lakehouse architecture, and feature store design—with real-time data science workflows to support personalization at scale.
What follows is a breakdown of its primary subsystems:
5.1.1. Data Ingestion: Data Ingestion, **in the context of Adaptive Content Optimization, refers to the process of collecting, streaming, and normalizing data from multiple sources—such as web/app analytics, CRM platforms, content systems, and third-party APIs—into a centralized pipeline. This enables real-time transformation into structured, ML-ready features. It supports both batch (ETL) and real-time (streaming) paradigms using frameworks like Kafka and Spark. Data Ingestion is foundational for feeding predictive models, segmentation algorithms, and content engines with fresh, high-fidelity signals at low latency.
5.1.1.1. What data to ingest
5.1.1.2. Where to store the data - Data Lake (Centralized Data Repository)
5.1.1.3. How to prepare the data for downstream - Data Processing (Real-time: Kafka/Flink; Batch: Spark)
5.1.1.4. How to bring or send the data to other parts of the system - Integration with Experience Orchestration Layer such as CMS/DAM Tools
Experience Orchestration Layer platforms—such as Content Management Systems (CMS) and Digital Asset Management (DAM)—are core components of modern digital experience architecture. CMS manages and delivers structured content across web and mobile channels, while DAM systems store, govern, and version multimedia assets. Together, they enable intelligent orchestration of modular, personalized content through APIs and real-time integrations with decisioning and ML pipelines.
In Adaptive Content Optimization, the CMS/DAM layer serves as the final content rendering engine and is tightly coupled with the feature store and decisioning layer. APIs, gRPC streams, or Kafka topics enable real-time data interchange between ML services and front-end delivery infrastructure.
5.2. Audience Analytics Engine: This engine forms the analytical core of Adaptive Content Optimization, where real-time decisions are made based on enriched user signals, predictive modeling, and optimization logic. By combing AI/ML algorithms with business rules, the Audience Analytics Engine transforms static content delivery into dynamic, context-aware personalization pipelines.
The Audience Analytics Engine interprets features ingested from upstream layers, segments audiences, scores intent and likelihood, and determines the most optimal audience to target — all within milliseconds.
Let us look at some most important components of Audience Analytics Engine:
5.2.1. Micro-Segmentation: User micro-segmentation is the process of dividing users into fine-grained, behaviorally and contextually relevant clusters. This enables Hyper-personalized content delivery tailored to niche audience subsets.
In Adaptive Content Optimization, this segmentation is typically achieved using unsupervised learning techniques such as:
These clustering techniques operate on high-dimensional feature vectors that may include browsing history, purchase recency, geo-location, and device usage. Prior to clustering, feature engineering techniques such as PCA (Principal Component Analysis) is often applied to reduce dimensionality while preserving variance.
The outputs of clustering serve as latent segments that feed downstream into content recommendation engines, lookalike modeling, and reinforcement learning agents—each adapting messaging to the unique preferences and context of each micro-segment.
5.2.2. Propensity Modeling (Action Prediction: Clicks, Purchases, Subscriptions) and Look Alike Audience Modeling
Propensity modeling is a form of supervised learning that estimates the probability of a specific user action—such as clicking an ad, subscribing to a service, or making a purchase—based on historical data.
As data scientists, we train these models on labeled datasets using algorithms like logistic regression, random forests, or gradient boosting (e.g., XGBoost, LightGBM) to generate actionable scores between 0 and 1, representing the likelihood of conversion. These scores allow marketers to segment users not only by who they are, but by what they are likely to do next, enabling proactive engagement strategies such as personalized offers, retargeting, and tailored user journeys.
Lookalike (LAL) modeling builds upon this by identifying new users who share behavioral and demographic similarities with high-converting cohorts. This is typically done using unsupervised clustering followed by supervised similarity ranking. These models are especially valuable for scaling acquisition campaigns while maintaining efficiency.
Together, propensity and lookalike modeling turn historical data into predictive intelligence that drives revenue and marketing ROI.
5.2.3. Predictive Analytics to predict key outcomes (like click probability or conversion likelihood) using Logistic Regression, Gradient Boosting (e.g., XGBoost)
For business stakeholders, predictive analytics transforms historical and real-time data into forward-looking insights that inform high-value decisions, such as which segment to retarget or which user journey to prioritize.
From a data science perspective, these models rely on labeled datasets, where past user actions serve as training signals.
For example, if you’re building a model to predict whether a user will click on an ad, your labeled dataset would include features like device type, time of day, page context, and user behavior history—alongside a binary label:
if they clicked,
1 -
0 -
if they didn’t.
Typical labeled datasets in adaptive content optimization may include:
Below are several widely used machine learning algorithms for classification and scoring tasks:
Feature engineering plays a critical role in improving model signal. Features may include recency, frequency, session depth, prior campaign interaction, device type, and sentiment extracted from clickstream or CRM data.
Once trained, these models generate predictive scores which are integrated into campaign logic—such as boosting high-propensity users into priority audiences or suppressing users likely to churn. These predictions are refreshed through batch or real-time pipelines depending on the frequency of user interactions.
5.3. Dynamic Content Generation Engine using AI models such Large Language Models (LLMs) fine tuned on domain specific data
This is the brain behind Adaptive Content Optimization—an intelligent content generation engine that uses fine-tuned Large Language Models (LLMs) to dynamically craft hyper-personalized content such as landing pages and ad copy. By integrating contextual signals, domain-specific knowledge, and user behavior data, this system generates marketing assets in real time, optimized for engagement and conversion. It serves as the decision and creation layer within the ACO architecture, transforming raw user and campaign signals into adaptive, high-performance content.
Traditional rule-based personalization systems rely heavily on predefined templates, manual copywriting, and A/B testing, which do not scale effectively. LLMs offer a generative approach where:
Foundation LLM models such as GPT-4.5, LLaMA, Claude are pre-trained on massive internet-scale corpora to develop general language capabilities, but this broad training lacks the precision needed for domain-specific applications in digital marketing.
We Fine-tune these models with high-quality, domain-specific data to enable these models to specialize in brand voice, tone, and content formats that align with key performance goals.
Domain-specific datasets may include:
Tuning the LLM on this curated content allows it to:
This process bridges the gap between general-purpose LLM capabilities and the nuanced demands of conversion-focused content generation.
Fine-tuning techniques transform general-purpose LLMs into highly specialized marketing content generators. Each technique addresses unique trade-offs between latency, cost, data availability, and model adaptability:
Fine-tuned LLMs are emerging as key infrastructure for high-performance digital marketing. When deployed within an end-to-end MLOps stack, they enable dynamic content generation that is real-time, adaptive, and business-aware.
5.4. Dynamic Content Assembly and Presentation Algorithms
Dynamic Content Assembly orchestrates the dynamic assembly of content across web, mobile, and ad delivery systems.
5.4.1. Modular Content Repository
5.4.2. Real-Time Content Assembler and Presentation
At the core of the Adaptive Content Optimization architecture are Dynamic Content Presentation Algorithms that map the output of Audience Analytics Engine with the output of Dynamic Content Generation Algorithm to serve the Right content to the right user to optimize for a business outcome.
This start with defining the Business outcome. It could be click-through rate (CTR), conversion rate (CVR), or return on ad spend (ROAS).
Dynamic Content Presentation Algorithms, then, leverages LLM-generated outputs and apply performance-based heuristics and machine learning techniques to optimize toward the predefined business outcomes.
Key approaches include:
These algorithms form the bridge between generation and delivery—continuously learning from user interactions, updating content strategies in real-time, and ensuring that each content variant is optimized for business outcomes.
5.5. Business Rule Engine (Brand, Ethical, Regulatory Compliance)
In addition to machine learning-driven personalization, many a times we need to provide an override for human intervention or manual quality check. The business rule engine is usually responsible for enforcing non-negotiable brand, ethical, and regulatory constraints. These rules ensure that even dynamically generated content adheres to legal, design, and cultural guidelines.
Key functions include:
This logic engine operates alongside the optimization algorithms and content generation layers, acting as a guardrail mechanism to balance automation with risk management.
5.6. Feedback & Learning Loop
The Feedback & Learning Loop is critical to maintaining model accuracy, content relevance, and sustained campaign performance in dynamic environments. This system continuously gathers user interaction data and uses it to refine both predictive models and generative systems through structured MLOps pipelines.
5.6.1 Real-Time Feedback Capture
5.6.2 Adaptive Learning (Model Refinement)
This closed-loop architecture ensures that ACO platforms not only adapt to user behavior in real time but also evolve structurally to improve content targeting, optimize asset combinations, and sustain business outcomes over time.
5.7. Governance, Privacy, Compliance, and Hybrid Automated Quality Checks
ACO platforms operate in highly regulated, brand-sensitive environments. This layer ensures that content generation and delivery processes uphold data protection, legal standards, and brand integrity through automated and human-in-the-loop systems.
5.7.1 Privacy Management
5.7.2 Transparency & Accountability
5.7.3 Pre-Approved Assets and QA Automation
Together, these systems balance innovation and control—allowing rapid experimentation and dynamic personalization while ensuring safety, compliance, and brand fidelity in every customer interaction.
From an architectural standpoint, building and deploying an Adaptive Content Optimization (ACO) system requires a rigorous focus on production-readiness and operational robustness. This chapter highlights key non-functional aspects that any enterprise-scale ACO implementation must account for, including system latency, scalability, model drift, and explainability.
Latency: In an ACO platform, latency directly impacts user experience. In-session personalization must respond in under 150 milliseconds to be perceived as real-time. Architecturally, this necessitates:
Model Drift: As user behavior evolves, model performance degrades over time—a phenomenon known as model drift. Robust ACO platforms implement:
Scalability: The ACO infrastructure must elastically scale to handle peak user load, especially during high-traffic campaigns. Key components of scalable architecture include:
Explainability: Transparency in AI decisions is critical in both regulated industries and for stakeholder trust. ACO systems embed explainability at multiple levels:
By proactively addressing these technical dimensions, organizations can ensure their ACO platforms are not just accurate and performant, but also resilient, scalable, and trustworthy in high-stakes marketing environments.
------------------------------------------------------------------------------
As AI maturity accelerates, marketing teams will move beyond traditional automation toward fully autonomous systems that execute and optimize campaigns with minimal human input. The next frontier of Adaptive Content Optimization (ACO) will be defined by its ability to orchestrate experiences, not just optimize them.
Adaptive Content Optimization will evolve from a tactical layer to a strategic operating model. In this AI-native future:
Forward-thinking leaders must invest in:
ACO is not merely a tool. It is a dynamic, evolving intelligence layer that will underpin how modern brands engage, convert, and retain audiences in the years ahead.
CoMarketer ACO exemplifies this evolution. It is not a standalone utility but a strategic module within a broader AI capability suite tailored for digital marketing teams. As an integral component of the modern marketing operating system, CoMarketer ACO provides an intelligent decision-making layer that leverages continuous feedback loops, self-optimizing algorithms, and real-time inference to orchestrate individualized brand experiences.
AI in this context doesn’t simply automate repetitive workflows—it augments human strategy with predictive foresight, adaptive learning, and generative creativity. By analyzing behavioral telemetry, contextual signals, and business rules simultaneously, platforms like CoMarketer ACO empower marketers to refine targeting precision, optimize content delivery, and improve conversion economics at scale.
In this rapidly evolving digital ecosystem, organizations that operationalize AI-driven marketing strategies gain a sustainable competitive advantage. Intelligent orchestration ensures that every customer interaction is relevant, timely, and high-converting, enabling businesses to deliver personalization at scale without compromising brand coherence or compliance.
As AI continues to transform the martech landscape, companies that embed these intelligent systems at the core of their marketing infrastructure will be uniquely positioned to drive business growth, deepen customer relationships, and lead in the experience economy.
As a photographer, it’s important to get the visuals right while establishing your online presence. Having a unique and professional portfolio will make you stand out to potential clients. The only problem? Most website builders out there offer cookie-cutter options — making lots of portfolios look the same.
That’s where a platform like Webflow comes to play. With Webflow you can either design and build a website from the ground up (without writing code) or start with a template that you can customize every aspect of. From unique animations and interactions to web app-like features, you have the opportunity to make your photography portfolio site stand out from the rest.
So, we put together a few photography portfolio websites that you can use yourself — whether you want to keep them the way they are or completely customize them to your liking.
Here are 12 photography portfolio templates you can use with Webflow to create your own personal platform for showing off your work.
Subscribe to our newsletter to receive our latest blogs, recommended digital courses, and more to unlock growth Mindset
The objective of marketing has always been to show the right content to the right person at the right time. Historically, this ambition faced limitations due to manual segmentation, inconsistent user data, and limited delivery mechanisms. With the proliferation of digital touchpoints, achieving contextual relevance became even harder.
Traditional approaches rely heavily on rules-based personalization and static audience segmentation, where users are grouped into broad categories based on predefined criteria. These techniques lack the flexibility and granularity needed to engage today’s dynamic, always-on consumer.
Such systems often depend on historical averages and basic conditional logic, which fail to adapt to real-time behavioral signals or content performance feedback. The following limitations emerge:
To overcome these shortcomings, organizations need real time hyper-personalized adaptive systems that incorporate machine learning models, feedback mechanisms, and content intelligence engines to provide hyper-relevant, scalable, and cohesive user experiences across the customer journey.
Enters AI-powered Adaptive Content Optimization (ACO), a paradigm shift that merges real-time decision-making with machine learning to transform how content is created, delivered, and optimized across channels. This approach enables:
With adaptive content optimization, marketing becomes not only automated but also intelligent, iterative, and intimately connected to user intent and journey stage—enabling performance marketing at scale.
This new paradigm uses a suite of AI/ML models to detect intent, predict outcomes, and deliver dynamically composed experiences in real-time. Intent detection is powered by natural language understanding (NLU) and behavioral modeling using Transformer-based architectures (e.g., BERT, RoBERTa) which parse user interactions and classify them into specific intents or emotional states. Outcome prediction leverages supervised learning models such as Gradient Boosted Trees (e.g., XGBoost, LightGBM) and deep neural networks to score user actions like click-throughs or conversions with high precision.
For content generation and orchestration, reinforcement learning agents (e.g., Deep Q-Networks, Proximal Policy Optimization) continually adjust creative strategies based on real-time performance signals. Dynamic content assembly is guided by ranking models and contextual bandits, which balance exploration of new content with exploitation of known high-performing variants. These models are deployed in production using scalable inference platforms like TensorFlow Serving or ONNX Runtime, enabling millisecond-level decision-making.
Together, these systems enable marketers to algorithmically compose and serve content variants in response to each user’s real-time behavior, contextual conditions, and stage in the conversion funnel, creating a truly adaptive and responsive digital experience.
Let us look at two case studies of how we leveraged Machine Learning and Gen AI to deliver Hyper Personalized digital experiences through our Co-Marketer Platform to our end clients.
Case Study 2.1: NeutoAI’s AI-Driven Personalization Boosts Sales for a Leading Fashion Retailer
Client Overview
A well-known online fashion retailer faced high cart abandonment, low engagement, and declining conversion rates, despite a broad product catalog.
They engaged NeutoAI to implement an AI-driven solution that dynamically optimized content and created a hyper-personalized shopping experience.
GC developed and deployed CoMarketers, an advanced AI-powered personalization engine tailored to the client’s needs.
Solution & Implementation
1. AI-Driven User Behavior Tracking
2. Real-Time Personalization at Scale
When Jen returned to the site, the homepage dynamically updated to showcase:
3. AI-Powered Email & Push Notification Optimization
GC’s AI detected that Jen engages with emails in the evening and optimized messaging accordingly. She received a personalized email at 7 PM featuring:
📩 Subject Line: “Jen, Your Perfect Sneakers Are Waiting!”
🎯 A carousel of dynamic product recommendations based on her past searches.
💰 A special offer: “Complete Your Look – 10% Off Running Jackets for the Next 24 Hours!”
4. Real-Time Adjustments Based on Engagement
If Jen clicked on a product but didn’t purchase, the AI dynamically adapted her shopping experience:
🔹 AI-powered chat assistance recommended similar styles based on her preferences.
🔹 A pop-up alert created urgency: “Only 3 left in stock! Grab yours now.”
🔹 Social proof notifications boosted confidence: “150+ people bought this in the last 24 hours.”
Results & Impact
📈 28% increase in funnel conversion rates over 6 months.
🛒 20% reduction in cart abandonment after personalized retargeting efforts.
📧 35% higher engagement with AI-personalized emails compared to generic campaigns.
By implementing NeutoAI’s AI-powered dynamic content optimization, the retailer transformed its digital shopping experience—ensuring every customer interaction was timely, relevant, and conversion-driven. 🚀
Case Study 2.2: NeutoAI’s AI-Driven Personalization Enhances Telecom Plan Selection
Client Overview
A prominent telecom provider approached NeutoAI with challenges including low online conversions, frequent user drop-offs, and decreased engagement, despite offering an extensive range of telecom plans. To address these challenges, they partnered with NeutoAI to deploy an advanced AI-driven personalization strategy designed to dynamically optimize content and deliver a highly personalized user experience.
GrowthClap developed and implemented CoMarketers, a sophisticated AI-powered personalization engine tailored specifically to the telecom provider’s unique needs.
Solution & Implementation
1. AI-Driven User Behavior Tracking
A user, Sharad, visited the telecom website and explored unlimited data plans but exited without subscribing. Sharad, during his visit to the website, also reviewed family plans and compared various international calling options, demonstrating clear intent but not finalizing his decision.
GrowthClap’s AI system captured Sharad’s browsing behaviors, plan preferences, and potential purchase intentions in real time.
2. Real-Time Landing Page Personalization at Scale
Upon Sharad’s return to the telecom website, the homepage was dynamically adjusted through CoMarketers to display:
3. AI-Powered Email & Push Notification Optimization
Leveraging CoMarketers’ AI analytics, NeutoAI determined that Sharad typically engages more actively with marketing emails during morning hours.
4. Real-Time Adjustments Based on Engagement
If Sharad interacted with a product but still did not complete the purchase, CoMarketers immediately adjusted his experience:
🔹 An AI-powered chatbot proactively offered Sharad a personalized plan quiz to match telecom services accurately with his usage patterns.
🔹 A strategically timed limited-time pop-up appeared, stating: “Exclusive: 20% off your first 3 months if you switch today!” to encourage immediate action.
🔹 Social proof notifications reassured his choice by displaying messages like “5,000+ users switched to this plan last month!”, increasing the likelihood of purchase.
Results & Impact
📈 Significant increase in online plan conversions due to highly relevant personalized interactions.
🛒 Reduced abandonment rate after deployment of targeted real-time retargeting strategies.
📧 Higher email engagement, with substantial improvements compared to generic email campaigns.
By implementing NeutoAI’s sophisticated AI-powered dynamic content optimization, the telecom provider substantially improved user experience, ensuring each interaction was personalized, timely, and conducive to conversion. 🚀
These are prime examples of AI-driven hyper-personalization, ensuring end customers are presented with the right content at the right time based on their expressed or latent needs.
In this article, We will dive deeper to understand how businesses can craft highly targeted campaigns, enhance customer experiences, and maximize return on investment (ROI) by leveraging Machine learning, Natural language processing (NLP), and Real-time data analytics.
1️⃣ Advertising & Marketing Campaigns
Use Case: AI-driven A/B testing dynamically adjusts ad creatives, headlines, and CTAs based on real-time engagement.
Example: A digital ad campaign automatically switches to a high-performing version when click-through rates (CTR) drop below a threshold.
2️⃣ E-Commerce & Product Recommendations
Use Case: Personalized shopping experiences with dynamically optimized product pages.
Example: An online retailer adjusts homepage banners and product recommendations based on browsing behavior and past purchases.
3️⃣ Email & Push Notification Personalization
Use Case: AI optimizes subject lines, content, and send times to maximize open rates and engagement.
Example: A travel app sends personalized hotel deals based on a user’s recent searches and preferences.
4️⃣ Content Streaming & Media Personalization
Use Case: Platforms adapt content based on viewing behavior and engagement patterns.
Example: A music streaming app dynamically updates playlists based on listening habits, time of day, or mood.
5️⃣ Website & Landing Page Optimization
Use Case: Real-time content customization based on visitor behavior and demographics.
Example: A B2B SaaS company dynamically adjusts case studies shown on the website based on industry and user location.
6️⃣ Conversational AI & Chatbots
Use Case: AI-powered chatbots modify their responses based on user sentiment, past interactions, and browsing history.
Example: An AI assistant for a telecom company adapts its responses to recommend personalized phone plans.
7️⃣ Retail In-Store Digital Displays
Use Case: Adaptive digital signage changes promotions and content based on customer demographics and store traffic patterns.
Example: A clothing store display showcases relevant outfits based on local weather conditions and sales trends.
8️⃣ Customer Support & Knowledge Base Optimization
Use Case: AI dynamically adjusts FAQs and suggested help articles based on user queries.
Example: A software company’s help center prioritizes troubleshooting guides that match user behavior and past support tickets.
9️⃣ Social Media Content Optimization
Use Case: AI adapts post formats, hashtags, and imagery based on audience engagement trends.
Example: A fashion brand’s Instagram posts are automatically optimized based on trending styles and user engagement.
ACO is powered by a synergy of advanced AI/ML components and infrastructure, enabling real-time, hyper-personalized content experiences at scale:
Together, these components form a robust adaptive content pipeline capable of ingesting real-time signals, making intelligent decisions at millisecond latency, and delivering contextually aware experiences that evolve continuously with user behavior and business objectives.
Before we explore each architectural component in depth, it’s important to understand the overarching objective of the ACO architecture: to seamlessly connect real-time user data with decision engines, dynamic content delivery systems, and adaptive learning mechanisms in a way that is both scalable and responsive.
At a high level, the ACO architecture is structured to process vast streams of data—both historical and real-time—using a combination of machine learning pipelines, streaming data frameworks, and API-based integrations. These layers work in concert to:
The architecture is modular and highly extensible, supporting integration with third-party marketing stacks, analytics platforms, content management systems, and experimentation tools.
The following sections provide a detailed breakdown of each architectural layer and the AI/ML models, technologies, and business logic underpinning them.
5.1. Data Integration and Data Management Layer
The Data Integration and Management Layer serves as the foundational backbone of any Adaptive Content Optimization (ACO) architecture. It is responsible for capturing, normalizing, enriching, and transforming a variety of high-volume, high-velocity data streams from digital sources into structured representations that power downstream machine learning pipelines and personalization engines.
This layer combines best practices from modern data engineering—such as event-driven stream ingestion, data lakehouse architecture, and feature store design—with real-time data science workflows to support personalization at scale.
What follows is a breakdown of its primary subsystems:
5.1.1. Data Ingestion: Data Ingestion, **in the context of Adaptive Content Optimization, refers to the process of collecting, streaming, and normalizing data from multiple sources—such as web/app analytics, CRM platforms, content systems, and third-party APIs—into a centralized pipeline. This enables real-time transformation into structured, ML-ready features. It supports both batch (ETL) and real-time (streaming) paradigms using frameworks like Kafka and Spark. Data Ingestion is foundational for feeding predictive models, segmentation algorithms, and content engines with fresh, high-fidelity signals at low latency.
5.1.1.1. What data to ingest
5.1.1.2. Where to store the data - Data Lake (Centralized Data Repository)
5.1.1.3. How to prepare the data for downstream - Data Processing (Real-time: Kafka/Flink; Batch: Spark)
5.1.1.4. How to bring or send the data to other parts of the system - Integration with Experience Orchestration Layer such as CMS/DAM Tools
Experience Orchestration Layer platforms—such as Content Management Systems (CMS) and Digital Asset Management (DAM)—are core components of modern digital experience architecture. CMS manages and delivers structured content across web and mobile channels, while DAM systems store, govern, and version multimedia assets. Together, they enable intelligent orchestration of modular, personalized content through APIs and real-time integrations with decisioning and ML pipelines.
In Adaptive Content Optimization, the CMS/DAM layer serves as the final content rendering engine and is tightly coupled with the feature store and decisioning layer. APIs, gRPC streams, or Kafka topics enable real-time data interchange between ML services and front-end delivery infrastructure.
5.2. Audience Analytics Engine: This engine forms the analytical core of Adaptive Content Optimization, where real-time decisions are made based on enriched user signals, predictive modeling, and optimization logic. By combing AI/ML algorithms with business rules, the Audience Analytics Engine transforms static content delivery into dynamic, context-aware personalization pipelines.
The Audience Analytics Engine interprets features ingested from upstream layers, segments audiences, scores intent and likelihood, and determines the most optimal audience to target — all within milliseconds.
Let us look at some most important components of Audience Analytics Engine:
5.2.1. Micro-Segmentation: User micro-segmentation is the process of dividing users into fine-grained, behaviorally and contextually relevant clusters. This enables Hyper-personalized content delivery tailored to niche audience subsets.
In Adaptive Content Optimization, this segmentation is typically achieved using unsupervised learning techniques such as:
These clustering techniques operate on high-dimensional feature vectors that may include browsing history, purchase recency, geo-location, and device usage. Prior to clustering, feature engineering techniques such as PCA (Principal Component Analysis) is often applied to reduce dimensionality while preserving variance.
The outputs of clustering serve as latent segments that feed downstream into content recommendation engines, lookalike modeling, and reinforcement learning agents—each adapting messaging to the unique preferences and context of each micro-segment.
5.2.2. Propensity Modeling (Action Prediction: Clicks, Purchases, Subscriptions) and Look Alike Audience Modeling
Propensity modeling is a form of supervised learning that estimates the probability of a specific user action—such as clicking an ad, subscribing to a service, or making a purchase—based on historical data.
As data scientists, we train these models on labeled datasets using algorithms like logistic regression, random forests, or gradient boosting (e.g., XGBoost, LightGBM) to generate actionable scores between 0 and 1, representing the likelihood of conversion. These scores allow marketers to segment users not only by who they are, but by what they are likely to do next, enabling proactive engagement strategies such as personalized offers, retargeting, and tailored user journeys.
Lookalike (LAL) modeling builds upon this by identifying new users who share behavioral and demographic similarities with high-converting cohorts. This is typically done using unsupervised clustering followed by supervised similarity ranking. These models are especially valuable for scaling acquisition campaigns while maintaining efficiency.
Together, propensity and lookalike modeling turn historical data into predictive intelligence that drives revenue and marketing ROI.
5.2.3. Predictive Analytics to predict key outcomes (like click probability or conversion likelihood) using Logistic Regression, Gradient Boosting (e.g., XGBoost)
For business stakeholders, predictive analytics transforms historical and real-time data into forward-looking insights that inform high-value decisions, such as which segment to retarget or which user journey to prioritize.
From a data science perspective, these models rely on labeled datasets, where past user actions serve as training signals.
For example, if you’re building a model to predict whether a user will click on an ad, your labeled dataset would include features like device type, time of day, page context, and user behavior history—alongside a binary label:
if they clicked,
1 -
0 -
if they didn’t.
Typical labeled datasets in adaptive content optimization may include:
Below are several widely used machine learning algorithms for classification and scoring tasks:
Feature engineering plays a critical role in improving model signal. Features may include recency, frequency, session depth, prior campaign interaction, device type, and sentiment extracted from clickstream or CRM data.
Once trained, these models generate predictive scores which are integrated into campaign logic—such as boosting high-propensity users into priority audiences or suppressing users likely to churn. These predictions are refreshed through batch or real-time pipelines depending on the frequency of user interactions.
5.3. Dynamic Content Generation Engine using AI models such Large Language Models (LLMs) fine tuned on domain specific data
This is the brain behind Adaptive Content Optimization—an intelligent content generation engine that uses fine-tuned Large Language Models (LLMs) to dynamically craft hyper-personalized content such as landing pages and ad copy. By integrating contextual signals, domain-specific knowledge, and user behavior data, this system generates marketing assets in real time, optimized for engagement and conversion. It serves as the decision and creation layer within the ACO architecture, transforming raw user and campaign signals into adaptive, high-performance content.
Traditional rule-based personalization systems rely heavily on predefined templates, manual copywriting, and A/B testing, which do not scale effectively. LLMs offer a generative approach where:
Foundation LLM models such as GPT-4.5, LLaMA, Claude are pre-trained on massive internet-scale corpora to develop general language capabilities, but this broad training lacks the precision needed for domain-specific applications in digital marketing.
We Fine-tune these models with high-quality, domain-specific data to enable these models to specialize in brand voice, tone, and content formats that align with key performance goals.
Domain-specific datasets may include:
Tuning the LLM on this curated content allows it to:
This process bridges the gap between general-purpose LLM capabilities and the nuanced demands of conversion-focused content generation.
Fine-tuning techniques transform general-purpose LLMs into highly specialized marketing content generators. Each technique addresses unique trade-offs between latency, cost, data availability, and model adaptability:
Fine-tuned LLMs are emerging as key infrastructure for high-performance digital marketing. When deployed within an end-to-end MLOps stack, they enable dynamic content generation that is real-time, adaptive, and business-aware.
5.4. Dynamic Content Assembly and Presentation Algorithms
Dynamic Content Assembly orchestrates the dynamic assembly of content across web, mobile, and ad delivery systems.
5.4.1. Modular Content Repository
5.4.2. Real-Time Content Assembler and Presentation
At the core of the Adaptive Content Optimization architecture are Dynamic Content Presentation Algorithms that map the output of Audience Analytics Engine with the output of Dynamic Content Generation Algorithm to serve the Right content to the right user to optimize for a business outcome.
This start with defining the Business outcome. It could be click-through rate (CTR), conversion rate (CVR), or return on ad spend (ROAS).
Dynamic Content Presentation Algorithms, then, leverages LLM-generated outputs and apply performance-based heuristics and machine learning techniques to optimize toward the predefined business outcomes.
Key approaches include:
These algorithms form the bridge between generation and delivery—continuously learning from user interactions, updating content strategies in real-time, and ensuring that each content variant is optimized for business outcomes.
5.5. Business Rule Engine (Brand, Ethical, Regulatory Compliance)
In addition to machine learning-driven personalization, many a times we need to provide an override for human intervention or manual quality check. The business rule engine is usually responsible for enforcing non-negotiable brand, ethical, and regulatory constraints. These rules ensure that even dynamically generated content adheres to legal, design, and cultural guidelines.
Key functions include:
This logic engine operates alongside the optimization algorithms and content generation layers, acting as a guardrail mechanism to balance automation with risk management.
5.6. Feedback & Learning Loop
The Feedback & Learning Loop is critical to maintaining model accuracy, content relevance, and sustained campaign performance in dynamic environments. This system continuously gathers user interaction data and uses it to refine both predictive models and generative systems through structured MLOps pipelines.
5.6.1 Real-Time Feedback Capture
5.6.2 Adaptive Learning (Model Refinement)
This closed-loop architecture ensures that ACO platforms not only adapt to user behavior in real time but also evolve structurally to improve content targeting, optimize asset combinations, and sustain business outcomes over time.
5.7. Governance, Privacy, Compliance, and Hybrid Automated Quality Checks
ACO platforms operate in highly regulated, brand-sensitive environments. This layer ensures that content generation and delivery processes uphold data protection, legal standards, and brand integrity through automated and human-in-the-loop systems.
5.7.1 Privacy Management
5.7.2 Transparency & Accountability
5.7.3 Pre-Approved Assets and QA Automation
Together, these systems balance innovation and control—allowing rapid experimentation and dynamic personalization while ensuring safety, compliance, and brand fidelity in every customer interaction.
From an architectural standpoint, building and deploying an Adaptive Content Optimization (ACO) system requires a rigorous focus on production-readiness and operational robustness. This chapter highlights key non-functional aspects that any enterprise-scale ACO implementation must account for, including system latency, scalability, model drift, and explainability.
Latency: In an ACO platform, latency directly impacts user experience. In-session personalization must respond in under 150 milliseconds to be perceived as real-time. Architecturally, this necessitates:
Model Drift: As user behavior evolves, model performance degrades over time—a phenomenon known as model drift. Robust ACO platforms implement:
Scalability: The ACO infrastructure must elastically scale to handle peak user load, especially during high-traffic campaigns. Key components of scalable architecture include:
Explainability: Transparency in AI decisions is critical in both regulated industries and for stakeholder trust. ACO systems embed explainability at multiple levels:
By proactively addressing these technical dimensions, organizations can ensure their ACO platforms are not just accurate and performant, but also resilient, scalable, and trustworthy in high-stakes marketing environments.
------------------------------------------------------------------------------
As AI maturity accelerates, marketing teams will move beyond traditional automation toward fully autonomous systems that execute and optimize campaigns with minimal human input. The next frontier of Adaptive Content Optimization (ACO) will be defined by its ability to orchestrate experiences, not just optimize them.
Adaptive Content Optimization will evolve from a tactical layer to a strategic operating model. In this AI-native future:
Forward-thinking leaders must invest in:
ACO is not merely a tool. It is a dynamic, evolving intelligence layer that will underpin how modern brands engage, convert, and retain audiences in the years ahead.
CoMarketer ACO exemplifies this evolution. It is not a standalone utility but a strategic module within a broader AI capability suite tailored for digital marketing teams. As an integral component of the modern marketing operating system, CoMarketer ACO provides an intelligent decision-making layer that leverages continuous feedback loops, self-optimizing algorithms, and real-time inference to orchestrate individualized brand experiences.
AI in this context doesn’t simply automate repetitive workflows—it augments human strategy with predictive foresight, adaptive learning, and generative creativity. By analyzing behavioral telemetry, contextual signals, and business rules simultaneously, platforms like CoMarketer ACO empower marketers to refine targeting precision, optimize content delivery, and improve conversion economics at scale.
In this rapidly evolving digital ecosystem, organizations that operationalize AI-driven marketing strategies gain a sustainable competitive advantage. Intelligent orchestration ensures that every customer interaction is relevant, timely, and high-converting, enabling businesses to deliver personalization at scale without compromising brand coherence or compliance.
As AI continues to transform the martech landscape, companies that embed these intelligent systems at the core of their marketing infrastructure will be uniquely positioned to drive business growth, deepen customer relationships, and lead in the experience economy.